CTS Ontology Workshop 2023: Difference between revisions
mNo edit summary |
|||
(51 intermediate revisions by 3 users not shown) | |||
Line 1: | Line 1: | ||
<font size="+ | <font size="+2">Ontologies, AI and Electronic Health Records</font> | ||
<font size="+1">Feb 23 - 24, 2023 - Charleston, SC</font> | |||
<font size="+ | |||
[[File:Chs23sm.jpg|link=|right|alt=Charleston]] | [[File:Chs23sm.jpg|link=|right|alt=Charleston]] | ||
Line 12: | Line 10: | ||
'''The Clinical and Translational Science Ontology Group (CTSOG)''' invites you to join us February 23-24, 2023, in Charleston, SC to discuss the role of ontologies in improving electronic health records (EHR) systems by advancing semantic interoperability, translational research, and artificial intelligence (AI). As health data increases in volume and complexity, and artificial intelligence applications gain momentum, the need for careful planning and interoperability becomes more critical. The purpose of this workshop is to explore new paradigms in EHRs, by examining successes and failures of ontologies and AI in different areas of biomedicine and their role in equitable healthcare. | '''The Clinical and Translational Science Ontology Group (CTSOG)''' invites you to join us February 23-24, 2023, in Charleston, SC to discuss the role of ontologies in improving electronic health records (EHR) systems by advancing semantic interoperability, translational research, and artificial intelligence (AI). As health data increases in volume and complexity, and artificial intelligence applications gain momentum, the need for careful planning and interoperability becomes more critical. The purpose of this workshop is to explore new paradigms in EHRs, by examining successes and failures of ontologies and AI in different areas of biomedicine and their role in equitable healthcare. | ||
More about the '''[http://ncorwiki.buffalo.edu/index.php/Clinical_and_Translational_Science_Ontology_Group Clinical and Translational Science Ontology Group (CTSOG)]''' and previous meetings. | |||
== '''Themes''' == | == '''Themes''' == | ||
Line 59: | Line 59: | ||
* App-based parking next to 22 WestEdge building (signage with instructions posted onsite) | * App-based parking next to 22 WestEdge building (signage with instructions posted onsite) | ||
== ''' | =='''Registration'''== | ||
Registration is free. | |||
However, registration is required for planning purposes. Registration is now closed. | |||
== '''Agenda: Thursday Feb 23'''== | |||
'''08:30a''': Breakfast and Networking | |||
'''09:00a''': Introduction to the meeting | |||
''' | '''09:15-10:45a''' Working sessions/Presentations | ||
''' | *'''Barry Smith and Jobst Landgrebe:''' Discussion on AI and Medicine, with a special focus on ChatGPT | ||
Slides: [https://buffalo.box.com/v/Smith-on-ChatGPT Smith], [https://buffalo.box.com/v/Landgrebe-ChatGPT Landgrebe] | |||
[https://www. | [https://www.youtube.com/watch?v=2f5Dh56lOBY Video] | ||
[https:// | How are we to explain the peculiar tendency of ChatGPT to throw up what are called [https://buffalo.box.com/v/ChatGPT-Hallucinations ‘hallucinations’]? To answer this question we will draw on our book ''[https://buffalo.app.box.com/v/AI-Without-Fear Why Machines Will Never Rule the World]'', whose core thesis is that an artificial intelligence that could equal or exceed human intelligence—sometimes called "artificial general intelligence" (AGI)—is for mathematical reasons impossible. The argument for this thesis rests on the fact that (1.) human intelligence is a capability of a complex dynamic system—the human brain and central nervous system, and (2.) systems of this sort (like all organic systems) cannot be modelled mathematically in a way that would allow the models to operate inside a computer. We survey on this basis the potential of AI in the future of clinical and translational research. | ||
'''--break--''' | |||
'''11:00-12:30p''' Working sessions/Presentations | |||
*'''Discussion and brief presentations. Topics to include: | |||
:The Clinical and Translational Science Ontology Landscape in 2023 | |||
[https:// | [https://buffalo.box.com/s/6nefh88tsdmo0e1cuirprei2mq189wzn Video] | ||
'''12:30-01:30p''' Networking and Working Lunch | |||
'''01:30-3:00p''' Working sessions/Presentations | |||
*'''Yonghui Wu:''' A large language model for electronic health records. | |||
[https://buffalo.box.com/v/Yongui-AI-and-EHR Slides] | |||
Natural language processing (NLP) powered by pretrained language models is the key technology for medical AI systems utilizing clinical narratives. We develop from scratch a large clinical language model—GatorTron—using >90 billion words of text (including >82 billion words of de-identified clinical text). GatorTron models scale up the clinical language model from 110 million to 8.9 billion parameters and improve five clinical NLP tasks, which can be applied to medical AI systems to improve healthcare delivery. | |||
'''--break--''' | |||
''' | '''3:15-5:00p''' Working sessions/Presentations | ||
*'''Richard Ohrbach and Barry Smith:''' Defining 'Injury' | |||
[https://buffalo.box.com/v/Ohrbach-Defining-Injury Slides] | |||
[https://buffalo.box.com/s/tqjac00xph64t41q3i5lz00f2jskufxs Video] | |||
This session is intended as a contribution to the ontology component of a broader investigation of injury and pain. SNOMED-CT defines an injury as a 'disorder resulting from physical damage to the body'. The WHO defines an injury as a 'bodily lesion at the organic level, resulting from acute exposure to energy (mechanical, thermal, electrical, chemical or radiant), in amounts that exceed the threshold of physiological tolerance.' We will explore these and other definitions with a view to establishing a more coherent understanding of the ontology of injury and of related phenomena such as lesion, trauma, pain, and so forth. Further topics for possible discussion are listed <u>[[Injury | here]]</u>. For the ontology of pain, see in particular [https://philpapers.org/archive/SMITAO-12.pdf here] | |||
'''--break--''' | |||
'''5:30p''' Networking Reception | |||
''' | =='''Agenda: Friday Feb 24'''== | ||
- | '''08:30-09:15a''' Breakfast and Networking | ||
'''09:15-10:15a''' Working sessions/Presentations | |||
*''' | *'''William Hogan:''' Semantic Representation of Occupations as Social Determinants of Health | ||
[https://buffalo.box.com/v/Hogan-Occupations-Ontology Slides] | |||
[https://buffalo.box.com/s/tqjac00xph64t41q3i5lz00f2jskufxs Video] | |||
The ontological representation of occupation presents several challenges. For example, is it your occupation if you have not yet done it? Are you a nuclear engineer before you start your first job doing it? Also, if an occupation is a role (in the BFO sense), then how does it differ from a job role? If I have an occupation of professor, and have had four job roles as professors at different organizations, then that is one occupation numerically but four job roles. How to handle non-employment situations such as "retired" and "homemaker"? What if you concurrently hold two jobs in different "occupations"? I will discuss these issues in the context of recent discussions at the OMRSE monthly meeting and present some options for ontological representation of occupation and related entities. | |||
'''--break--''' | |||
'''10:30-12:30p''' Working sessions/Presentations | |||
- | *'''Justin Reese:''' Unsupervised machine learning to define subtypes of long COVID using the Human Phenotype Ontology] [[Authors | Co-authors]] | ||
[https://docs.google.com/presentation/d/1WKvoNl6yqiA00zH5qHkRfDKTksboTX897zU7yJpT8yY/edit#slide=id.g1dd10f2c7e8_0_4793 Slides] | |||
[https://buffalo.box.com/s/it19e7j0o26g5tgy64o6snm66944eyw7 Video] | |||
Stratification of patients with post-acute sequelae of SARS-CoV-2 infection (PASC, or long COVID) would allow precision clinical management strategies. However, long COVID is incompletely understood and characterized by a wide range of manifestations that are difficult to analyze computationally. Additionally, the generalisability of machine learning classification of COVID-19 clinical outcomes has rarely been tested. | |||
We present a method for defining subtypes of long COVID by computationally modeling PASC phenotype data and applying unsupervised machine learning. We extracted Long COVID phenotype data expressed as HPO (Human Phenotype Ontology) terms from electronic healthcare records (EHRs), and then used semantic similarity of phenotype data to calculate a matrix of pairwise patient similarity. This matrix was then used to clustered patients using unsupervised machine learning (k means clustering). | We present a method for defining subtypes of long COVID by computationally modeling PASC phenotype data and applying unsupervised machine learning. We extracted Long COVID phenotype data expressed as HPO (Human Phenotype Ontology) terms from electronic healthcare records (EHRs), and then used semantic similarity of phenotype data to calculate a matrix of pairwise patient similarity. This matrix was then used to clustered patients using unsupervised machine learning (k means clustering). | ||
Line 139: | Line 148: | ||
Semantic phenotypic clustering provides a foundation for assigning patients to stratified subgroups for natural history or therapy studies on PASC. | Semantic phenotypic clustering provides a foundation for assigning patients to stratified subgroups for natural history or therapy studies on PASC. | ||
12:30-01:30p Networking and Working Lunch | *'''Paul Heider:''' Beyond Structure: Using Standardized Labels from a Clinical Section Ontology in Natural Language Processing. | ||
[https://buffalo.box.com/v/Cllinical-Section-Ontology Slides] | |||
[https://buffalo.box.com/s/w4n2drw17v13imdc026flj5y5gqize2l Video] | |||
The structured subdivision of clinical notes into sections is often treated as the primary and ultimate use for automatically tagging section boundaries. We discuss how section information (especially when normalized to a standard ontology) can be used to augment natural language processing algorithms, simplify information retrieval tasks,and speed up system development through disaggregated performance evaluation. Our examples emphasize a Clinical Section Ontology recently developed at MUSC but are applicable to any standardized section ontology. We also include a brief overview of the annotated datasets in this domain. | |||
'''12:30-01:30p''' Networking and Working Lunch | |||
'''1:00-03:15p''' Working sessions/Presentations from industry partners | |||
*'''Andy Lin: (VP Strategy, CTO | Mark III Systems)''' NVIDIA DGX H100 – The Premier AI Platform for Research and LLMs. | |||
[https://buffalo.box.com/v/Lin-NVIDIA-DGX-H100 Slides] | |||
[https://buffalo.box.com/s/v9irq9k9c31yvtn97s91aenvbqfebfri Video] | |||
With up to 4x training and up to 30x inferencing performance improvements for Large Language Models (LLMs) vs the already industry leading A100 GPU, the recently launched H100 GPU (Hopper) and its purpose-built DGX H100 AI platform are transforming what’s possible with Research and LLMs. In this session, we’ll dive into the DGX H100 platform and how you can get started or accelerate your work with NVIDIA and Mark III. | |||
*'''John Doole: (TriNetX, LLC)''' Medication Informatics at TriNetX: Powering Clinical Research with a Federated Real World Data Network. | |||
[https://buffalo.box.com/v/Charleston-TriNetX Slides] | |||
[https://buffalo.box.com/v/Charleston-TriNetX Video] | |||
In this talk we will highlight the importance of medication informatics in clinical research, particularly in the context of a federated network. The talk discusses the challenges of data standardization and interoperability and the benefits of using medication ontologies and classification systems to improve data quality and efficiency. Additionally, the talk presents TriNetX's medication framework and how natural language processing is used to extract medication details, showcasing examples of its implementation and a discussion of the advantages and disadvantages of using NLP in clinical research. | |||
'''03:15-3:30p''' Closing Remarks | |||
== '''Participants''' == | == '''Participants''' == | ||
* | * Alekseyenko, Alex, Medical University of South Carolina | ||
* | * Baker, Hamilton, Medical University of South Carolina | ||
* | * Chatterjee, Prosenjit, The Citadel | ||
* Doole, John, TriNetX | |||
* | * Heider, Paul, Medical University of South Carolina | ||
* Jobst | * Hogan, William, co-organizer, University of Florida | ||
* | * Hutchinson, Tom, University of Pennsylvania, Institute for BioInformatics | ||
* Anna Maria | * Kim, Wonhee, Visiting Professor, Medical University of South Carolina | ||
* Richard | * Landgrebe, Jobst, Cognotekt, Cologne | ||
* Justin | * Lin, Andy, NVIDIA | ||
* Richard | * Masci, Anna Maria, National Institute of Environmental Health Sciences (NIEHS) | ||
* Matt | * Obeid, Jihad, co-organizer, Medical University of South Carolina | ||
* Umit | * Ohrbach, Richard, University at Buffalo | ||
* Reese, Justin, Lawrence Berkeley National Laboratory | |||
* Scheuermann, Richard, J. Craig Venter Institute | |||
* Simpson, Kit, Medical University of South Carolina | |||
* Skowronek, Matt, TriNetX | |||
* Smith, Barry, co-organizer, University at Buffalo | |||
* Topaloglu, Umit, Center for Biomedical Informatics and Information Technology (CBIIT) National Cancer Institute | |||
* Wehbe, Ramsey, Medical University of South Carolina | |||
* Yonghui Wu, University of Florida | * Yonghui Wu, University of Florida | ||
* Zheng, Jie, University of Pennsylvania |
Latest revision as of 19:53, 16 November 2023
Ontologies, AI and Electronic Health Records
Feb 23 - 24, 2023 - Charleston, SC
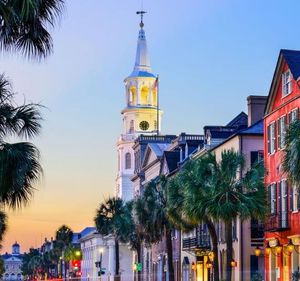
Background
The Clinical and Translational Science Ontology Group (CTSOG) invites you to join us February 23-24, 2023, in Charleston, SC to discuss the role of ontologies in improving electronic health records (EHR) systems by advancing semantic interoperability, translational research, and artificial intelligence (AI). As health data increases in volume and complexity, and artificial intelligence applications gain momentum, the need for careful planning and interoperability becomes more critical. The purpose of this workshop is to explore new paradigms in EHRs, by examining successes and failures of ontologies and AI in different areas of biomedicine and their role in equitable healthcare.
More about the Clinical and Translational Science Ontology Group (CTSOG) and previous meetings.
Themes
- Improving the EHR with ontologies and with AI
- The functions of the EHR and other healthcare documents
- Social Determinants of Health
- Mental health
- ChatGPT
Organizers
Workshop Co-organizers
Bill Hogan, Jihad Obeid, Barry Smith
CTSOG Co-chairs
Bill Hogan (University of Florida College of Medicine, Gainesville, FL), hoganwr@ufl.edu
Barry Smith (University at Buffalo, Buffalo, NY), phismith@buffalo.edu
Sponsors
- Medical University of South Carolina, the Biomedical Informatics Center and:
Venue
Biomedical Informatics Center
Medical University of South Carolina
Address: 22 Westedge St, Charleston, SC 29403
Directions: From Courtyard Marriott on Lockwood
- 10 minute walk, 0.4 miles, safe crosswalks along the route
- Hotel shuttle – runs periodically, need to ask hotel desk for timing details.
Paid parking:
- Parking deck at 10 WestEdge (building before 22 WestEdge, with Publix on bottom floor)
- App-based parking next to 22 WestEdge building (signage with instructions posted onsite)
Registration
Registration is free.
However, registration is required for planning purposes. Registration is now closed.
Agenda: Thursday Feb 23
08:30a: Breakfast and Networking
09:00a: Introduction to the meeting
09:15-10:45a Working sessions/Presentations
- Barry Smith and Jobst Landgrebe: Discussion on AI and Medicine, with a special focus on ChatGPT
How are we to explain the peculiar tendency of ChatGPT to throw up what are called ‘hallucinations’? To answer this question we will draw on our book Why Machines Will Never Rule the World, whose core thesis is that an artificial intelligence that could equal or exceed human intelligence—sometimes called "artificial general intelligence" (AGI)—is for mathematical reasons impossible. The argument for this thesis rests on the fact that (1.) human intelligence is a capability of a complex dynamic system—the human brain and central nervous system, and (2.) systems of this sort (like all organic systems) cannot be modelled mathematically in a way that would allow the models to operate inside a computer. We survey on this basis the potential of AI in the future of clinical and translational research.
--break--
11:00-12:30p Working sessions/Presentations
- Discussion and brief presentations. Topics to include:
- The Clinical and Translational Science Ontology Landscape in 2023
12:30-01:30p Networking and Working Lunch
01:30-3:00p Working sessions/Presentations
- Yonghui Wu: A large language model for electronic health records.
Natural language processing (NLP) powered by pretrained language models is the key technology for medical AI systems utilizing clinical narratives. We develop from scratch a large clinical language model—GatorTron—using >90 billion words of text (including >82 billion words of de-identified clinical text). GatorTron models scale up the clinical language model from 110 million to 8.9 billion parameters and improve five clinical NLP tasks, which can be applied to medical AI systems to improve healthcare delivery.
--break--
3:15-5:00p Working sessions/Presentations
- Richard Ohrbach and Barry Smith: Defining 'Injury'
This session is intended as a contribution to the ontology component of a broader investigation of injury and pain. SNOMED-CT defines an injury as a 'disorder resulting from physical damage to the body'. The WHO defines an injury as a 'bodily lesion at the organic level, resulting from acute exposure to energy (mechanical, thermal, electrical, chemical or radiant), in amounts that exceed the threshold of physiological tolerance.' We will explore these and other definitions with a view to establishing a more coherent understanding of the ontology of injury and of related phenomena such as lesion, trauma, pain, and so forth. Further topics for possible discussion are listed here. For the ontology of pain, see in particular here
--break--
5:30p Networking Reception
Agenda: Friday Feb 24
08:30-09:15a Breakfast and Networking
09:15-10:15a Working sessions/Presentations
- William Hogan: Semantic Representation of Occupations as Social Determinants of Health
The ontological representation of occupation presents several challenges. For example, is it your occupation if you have not yet done it? Are you a nuclear engineer before you start your first job doing it? Also, if an occupation is a role (in the BFO sense), then how does it differ from a job role? If I have an occupation of professor, and have had four job roles as professors at different organizations, then that is one occupation numerically but four job roles. How to handle non-employment situations such as "retired" and "homemaker"? What if you concurrently hold two jobs in different "occupations"? I will discuss these issues in the context of recent discussions at the OMRSE monthly meeting and present some options for ontological representation of occupation and related entities.
--break--
10:30-12:30p Working sessions/Presentations
- Justin Reese: Unsupervised machine learning to define subtypes of long COVID using the Human Phenotype Ontology] Co-authors
Stratification of patients with post-acute sequelae of SARS-CoV-2 infection (PASC, or long COVID) would allow precision clinical management strategies. However, long COVID is incompletely understood and characterized by a wide range of manifestations that are difficult to analyze computationally. Additionally, the generalisability of machine learning classification of COVID-19 clinical outcomes has rarely been tested.
We present a method for defining subtypes of long COVID by computationally modeling PASC phenotype data and applying unsupervised machine learning. We extracted Long COVID phenotype data expressed as HPO (Human Phenotype Ontology) terms from electronic healthcare records (EHRs), and then used semantic similarity of phenotype data to calculate a matrix of pairwise patient similarity. This matrix was then used to clustered patients using unsupervised machine learning (k means clustering).
We identified six clusters of PASC patients, each with distinct profiles of phenotypic abnormalities, including clusters with distinct pulmonary, neuropsychiatric, and cardiovascular abnormalities, and a cluster associated with broad, severe manifestations and increased mortality. There was significant association of cluster membership with a range of pre-existing conditions and measures of severity during acute COVID-19. We assigned new patients from other healthcare centers to clusters by maximum semantic similarity to the original patients, and showed that the clusters were generalisable across different hospital systems. The increased mortality rate originally identified in one cluster was consistently observed in patients assigned to that cluster in other hospital systems.
Semantic phenotypic clustering provides a foundation for assigning patients to stratified subgroups for natural history or therapy studies on PASC.
- Paul Heider: Beyond Structure: Using Standardized Labels from a Clinical Section Ontology in Natural Language Processing.
The structured subdivision of clinical notes into sections is often treated as the primary and ultimate use for automatically tagging section boundaries. We discuss how section information (especially when normalized to a standard ontology) can be used to augment natural language processing algorithms, simplify information retrieval tasks,and speed up system development through disaggregated performance evaluation. Our examples emphasize a Clinical Section Ontology recently developed at MUSC but are applicable to any standardized section ontology. We also include a brief overview of the annotated datasets in this domain.
12:30-01:30p Networking and Working Lunch
1:00-03:15p Working sessions/Presentations from industry partners
- Andy Lin: (VP Strategy, CTO | Mark III Systems) NVIDIA DGX H100 – The Premier AI Platform for Research and LLMs.
With up to 4x training and up to 30x inferencing performance improvements for Large Language Models (LLMs) vs the already industry leading A100 GPU, the recently launched H100 GPU (Hopper) and its purpose-built DGX H100 AI platform are transforming what’s possible with Research and LLMs. In this session, we’ll dive into the DGX H100 platform and how you can get started or accelerate your work with NVIDIA and Mark III.
- John Doole: (TriNetX, LLC) Medication Informatics at TriNetX: Powering Clinical Research with a Federated Real World Data Network.
In this talk we will highlight the importance of medication informatics in clinical research, particularly in the context of a federated network. The talk discusses the challenges of data standardization and interoperability and the benefits of using medication ontologies and classification systems to improve data quality and efficiency. Additionally, the talk presents TriNetX's medication framework and how natural language processing is used to extract medication details, showcasing examples of its implementation and a discussion of the advantages and disadvantages of using NLP in clinical research.
03:15-3:30p Closing Remarks
Participants
- Alekseyenko, Alex, Medical University of South Carolina
- Baker, Hamilton, Medical University of South Carolina
- Chatterjee, Prosenjit, The Citadel
- Doole, John, TriNetX
- Heider, Paul, Medical University of South Carolina
- Hogan, William, co-organizer, University of Florida
- Hutchinson, Tom, University of Pennsylvania, Institute for BioInformatics
- Kim, Wonhee, Visiting Professor, Medical University of South Carolina
- Landgrebe, Jobst, Cognotekt, Cologne
- Lin, Andy, NVIDIA
- Masci, Anna Maria, National Institute of Environmental Health Sciences (NIEHS)
- Obeid, Jihad, co-organizer, Medical University of South Carolina
- Ohrbach, Richard, University at Buffalo
- Reese, Justin, Lawrence Berkeley National Laboratory
- Scheuermann, Richard, J. Craig Venter Institute
- Simpson, Kit, Medical University of South Carolina
- Skowronek, Matt, TriNetX
- Smith, Barry, co-organizer, University at Buffalo
- Topaloglu, Umit, Center for Biomedical Informatics and Information Technology (CBIIT) National Cancer Institute
- Wehbe, Ramsey, Medical University of South Carolina
- Yonghui Wu, University of Florida
- Zheng, Jie, University of Pennsylvania